Learning Scientific Programming with Python (2nd edition)
E7.24: The two-dimensional diffusion equation
The two-dimensional diffusion equation is $$ \frac{\partial U}{\partial t} = D\left(\frac{\partial^2U}{\partial x^2} + \frac{\partial^2U}{\partial y^2}\right) $$ where $D$ is the diffusion coefficient. A simple numerical solution on the domain of the unit square $0\le x < 1, 0 \le y < 1$ approximates $U(x,y;t)$ by the discrete function $u_{i,j}^{(n)}$ where $x=i\Delta x$, $y=j\Delta y$ and $t=n\Delta t$. Applying finite difference approximations yields $$ \frac{u_{i,j}^{(n+1)} - u_{i,j}^{(n)}}{\Delta t} = D\left[ \frac{u_{i+1,j}^{(n)} - 2u_{i,j}^{(n)} + u_{i-1,j}^{(n)}}{(\Delta x)^2} + \frac{u_{i,j+1}^{(n)} - 2u_{i,j}^{(n)} + u_{i,j-1}^{(n)}}{(\Delta y)^2} \right], $$ and hence the state of the system at time step $n+1$, $u_{i,j}^{(n+1)}$ may be calculated from its state at time step $n$, $u_{i,j}^{(n)}$ through the equation $$ u_{i,j}^{(n+1)} = u_{i,j}^{(n)} + D\Delta t\left[ \frac{u_{i+1,j}^{(n)} - 2u_{i,j}^{(n)} + u_{i-1,j}^{(n)}}{(\Delta x)^2} + \frac{u_{i,j+1}^{(n)} - 2u_{i,j}^{(n)} + u_{i,j-1}^{(n)}}{(\Delta y)^2} \right]. $$
Consider the diffusion equation applied to a metal plate initially at temperature $T_\mathrm{cold}$ apart from a disc of a specified size which is at temperature $T_\mathrm{hot}$. We suppose that the edges of the plate are held fixed at $T_\mathrm{cool}$. The following code applies the above formula to follow the evolution of the temperature of the plate. It can be shown that the maximum time step, $\Delta t$ that we can allow without the process becoming unstable is $$ \Delta t = \frac{1}{2D}\frac{(\Delta x\Delta y)^2}{(\Delta x)^2 + (\Delta y)^2}. $$
In the code below, each call to do_timestep
updates the numpy array u
from the results of the previous timestep, u0
. The simplest approach to applying the partial difference equation is to use a Python loop:
for i in range(1, nx-1):
for j in range(1, ny-1):
uxx = (u0[i+1,j] - 2*u0[i,j] + u0[i-1,j]) / dx2
uyy = (u0[i,j+1] - 2*u0[i,j] + u0[i,j-1]) / dy2
u[i,j] = u0[i,j] + dt * D * (uxx + uyy)
However, this runs extremely slowly and using vectorization will farm out these explicit loops to the much faster pre-compiled C-code underlying NumPy's array implementation.
import numpy as np
import matplotlib.pyplot as plt
# plate size, mm
w = h = 10.0
# intervals in x-, y- directions, mm
dx = dy = 0.1
# Thermal diffusivity of steel, mm2.s-1
D = 4.0
Tcool, Thot = 300, 700
nx, ny = int(w / dx), int(h / dy)
dx2, dy2 = dx * dx, dy * dy
dt = dx2 * dy2 / (2 * D * (dx2 + dy2))
u0 = Tcool * np.ones((nx, ny))
u = u0.copy()
# Initial conditions - circle of radius r centred at (cx,cy) (mm)
r, cx, cy = 2, 5, 5
r2 = r**2
for i in range(nx):
for j in range(ny):
p2 = (i * dx - cx) ** 2 + (j * dy - cy) ** 2
if p2 < r2:
u0[i, j] = Thot
def do_timestep(u0, u):
# Propagate with forward-difference in time, central-difference in space.
u[1:-1, 1:-1] = u0[1:-1, 1:-1] + D * dt * (
(u0[2:, 1:-1] - 2 * u0[1:-1, 1:-1] + u0[:-2, 1:-1]) / dx2
+ (u0[1:-1, 2:] - 2 * u0[1:-1, 1:-1] + u0[1:-1, :-2]) / dy2
)
u0 = u.copy()
return u0, u
# Number of timesteps.
nsteps = 101
# Output 4 figures at these timesteps.
mfig = [0, 10, 50, 100]
fignum = 0
fig, axes = plt.subplots(nrows=2, ncols=2)
for m in range(nsteps):
u0, u = do_timestep(u0, u)
if m in mfig:
print(m, fignum)
ax = axes[fignum // 2, fignum % 2]
im = ax.imshow(
u.copy(),
cmap="hot",
vmin=Tcool,
vmax=Thot,
interpolation="bilinear",
)
ax.set_axis_off()
ax.set_title("{:.1f} ms".format(m * dt * 1000))
fignum += 1
fig.subplots_adjust(right=0.85)
cbar_ax = fig.add_axes([0.9, 0.15, 0.03, 0.7])
cbar_ax.set_xlabel("$T$ / K", labelpad=20)
fig.colorbar(im, cax=cbar_ax)
plt.show()
To set a common colorbar
for the four plots we define its own Axes
, cbar_ax
and make room for it with fig.subplots_adjust
. The plots all use the same colour range, defined by vmin
and vmax
, so it doesn't matter which one we pass in the first argument to fig.colorbar
.
The state of the system is plotted as an image at four different stages of its evolution.
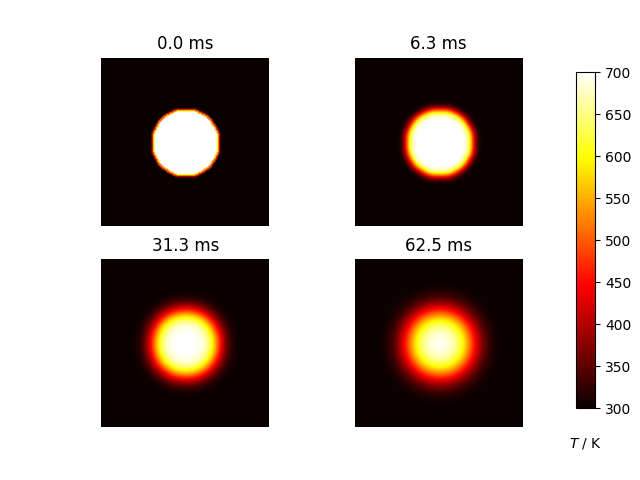
The two-dimensional diffusion equation.
Many thanks to Tim Teatro whose blog post inspired this example.